More well thought out work can be found at — https://axial.substack.com/
Axial invests and partners in early-stage life sciences companies. If you or someone you know has a great idea or company in life sciences, Axial would be excited to get to know you and possibly invest in your vision and company — info@axialsprawl.com
Observations #4
A set of ideas and observations from a week’s worth of work analyzing businesses and technologies.
AskBio business model
Similar to Regenxbio, AskBio is another great example of a vertically integrated gene therapy company. The company is led by the co-founder Sheila Mikhail (previously was the director of the Gene Therapy Center at the University of North Carolina) with major support from Jude Samulski who was the first to clone AAVs. The company has been around for almost 20 years (2001) - AskBio is a great example of a founder-driven life sciences company. The founders were not willing to sell ownership and control of their company ($1M on $1M pre-money). They had to run straight into gusting winds to achieve their goals. The medical community was skeptical of gene therapies, venture capital thought the businesses wouldn’t work, and biopharma didn’t believe in the business model. After 20 years, AskBio has played a major role to prove these 3 points wrong. Similar to Regenxbio, AskBio focused initially on ingesting AAV gene therapy IP. However, the latter decided to take a more active role with the companies they license their technology platform to. Whereas Regenxbio initially licensed their technology, AskBio almost similar to an incubator for AAV gene therapies. AskBio internally develops a drug and if there are early signs of success, spins the assets along with AAV IP and their corresponding manufacturing technologies off into a new company, which they call a special purpose entity (SPE). This model has enabled the founders to retain significant ownership and be cash-flow positive for some periods of time. With this model, the company has been able to spin out Chatham Therapeutics and Bamboo Therapeutics, which were acquired by Baxter and Pfizer, respectively. What makes AAV really amenable to this expansive platform model is due to their power - AAVs can be engineered (based on serotypes and pseudotyping) to be delivered to specific tissues and designed with precise expression of the cargo. This holding company approach could be more attractive to founders who want to focus on technology development. This is due to the biphasic nature of drug development - technologists don’t make the best drug developers:
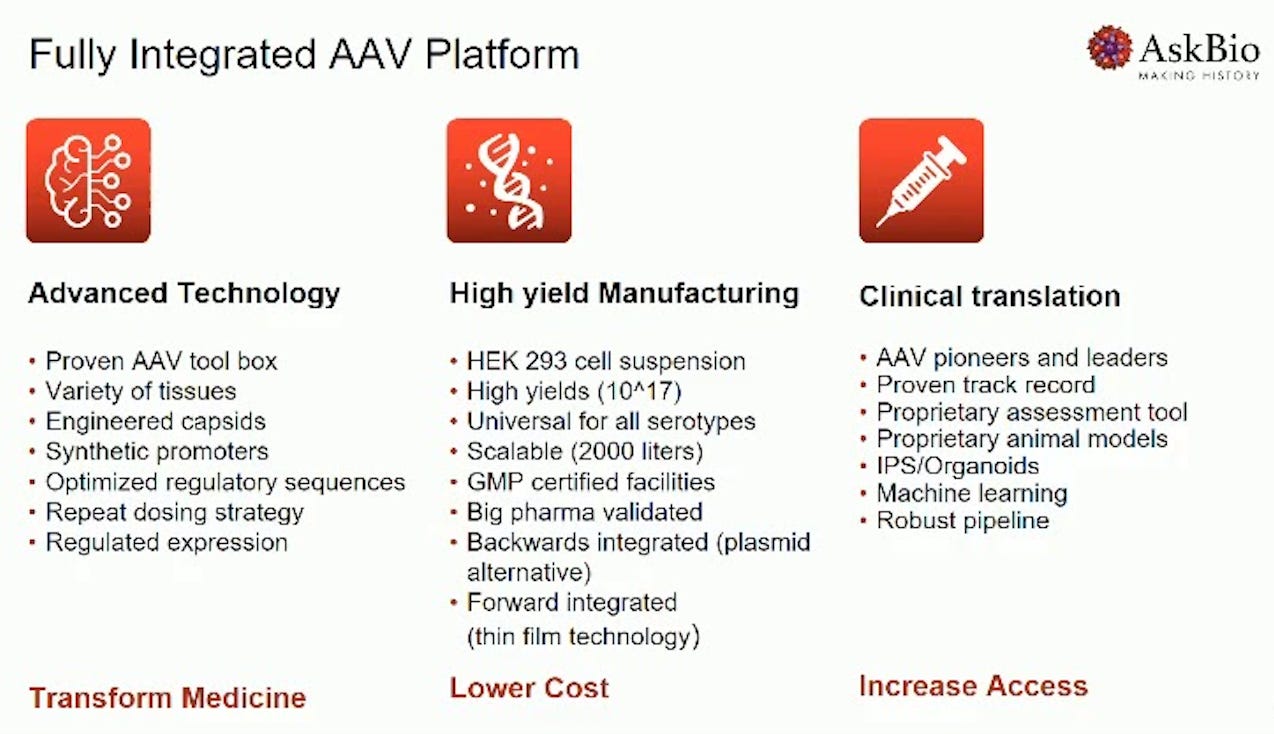
Source: AskBio
Design
The two major components of any AAV gene therapy are the capsid and delivery cargo including the flanking materials. AskBio is working with Touchlight Genetics to translate doggybone DNA (dbDNA), closed-linear, double stranded DNA, to replace traditional plasmid particularly antibiotic resistant genes and the need for large plasmid reactor volumes. These self-complementary vectors might help AskBio speed up the process of development. Moreover, AskBio is designing synthetic promoters to optimize transgene expression for a given tissue or cell type or a mode of delivering everything from constitutive, inducible, or active in a specific disease state. Their recent acquisition of Synpromics was a way to gain access to a larger library of promoters.
Manufacturing
With their design process, AskBio focused on vector manufacturing from day one - the process is the product. AskBio used a suspension-adapted HEK293 cell-line to produce their AAVs (up to 2K liters). The cell-lines maximize viral titers (i.e. produce fewer empty capsids) and minimize upfront development costs by being a transient transfection system (i.e. produce serotypes and chimeric AAVs).
Overall, there are 4 platforms for manufacturing AAV gene therapies:
Transient transfection of DNA in HEK293 cells (i.e. AskBio)
Recombinant baculovirus infection of insect cells
Adenovirus infection of cell lines of Rep/Cap plasmids co-infected with the AAV genome
Infecting the cell lines with two recombinant viruses - rHSVs and rHSV with Rep/Cap genes
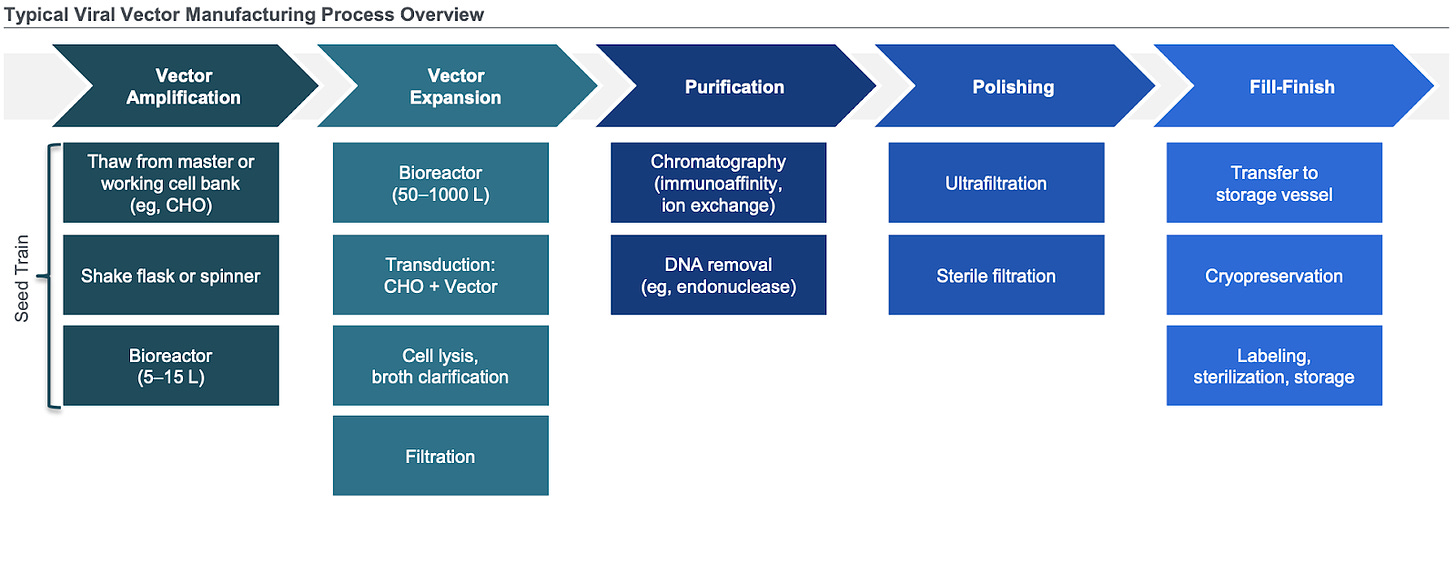
Source: Piper Jaffray
Acquisitions
In addition to the incubation model, AskBio has used its increasing war chest to acquire companies to build stronger moats around their AAV gene therapy design and manufacturing platform:
Synpromics - August 2019 - https://www.synpromics.com/news-media/askbio-acquires-synpromics-ltd-and-expands-its-gene-therapy-technology-portfolio
RoverMed BioSciences - September 2019 - https://www.biospace.com/article/releases/askbio-acquires-assets-from-rovermed-biosciences-adds-nanotechnology-precision-to-delivery-of-aav-gene-therapeutics/
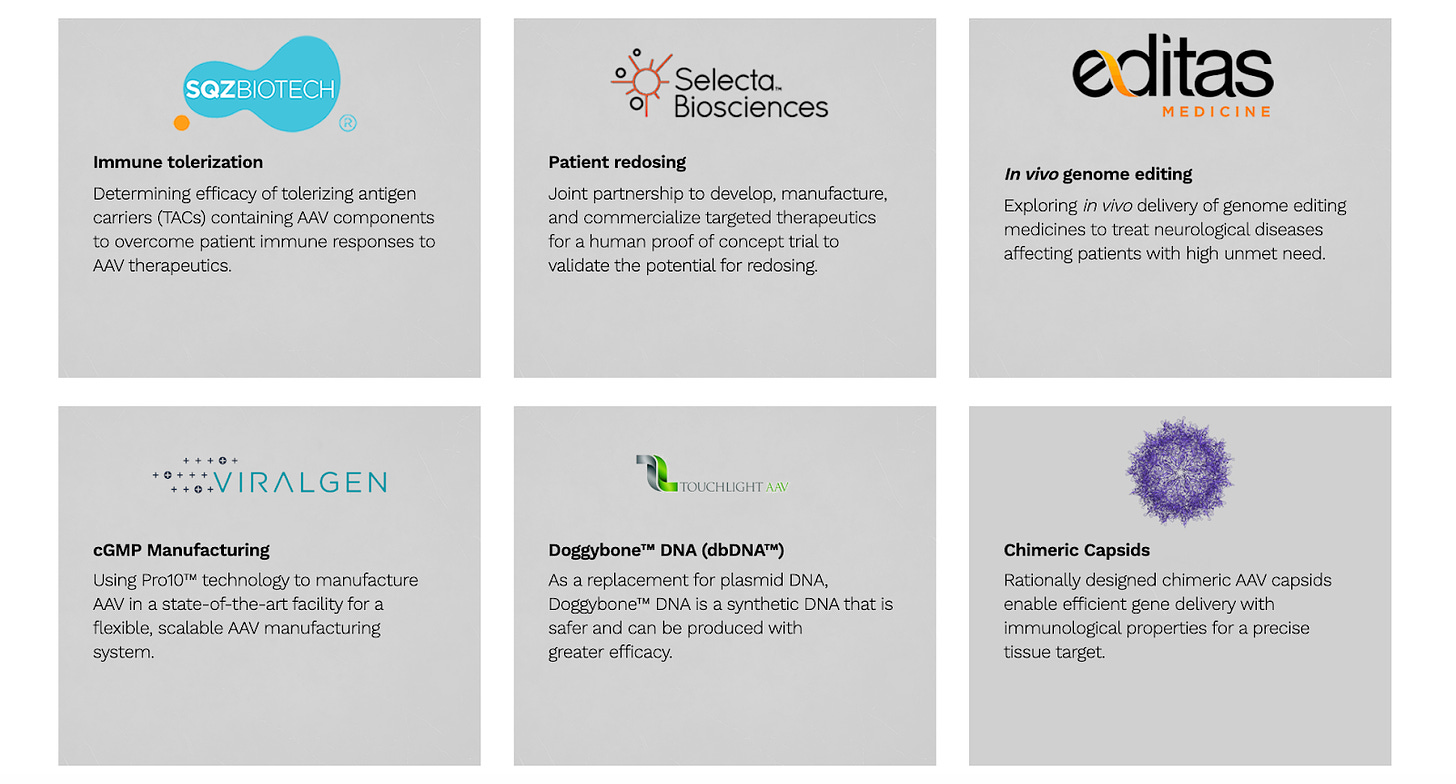
Source: AskBio
AskBio pioneered an incubation model for drug development. What’s exciting is the next steps. Can they successfully pursue pathway diseases? What about the post-clinical business model? Gene therapies and all curative medicines require longer follow ups whether the therapy is successful or not - this is not true for small molecules or biologics.
Productivity in biopharma
Drug development is becoming bottom heavy. Large biopharma companies are becoming less productive while the smaller ones are becoming more productive - https://www.nature.com/articles/d41573-019-00046-3 In the 1990s, the top 10 drug developers accounted for well more than half of all approved drugs and sponsored clinical trials. Over the last few years the 11-20 drug companies and especially the smaller companies are outpacing the top 10 (image below). This fragmentation is a strong indicator that monopolies in drug development are rare and maybe not possible. Whereas in other industries like search or e-commerce and even Coke, a company can own most of the market. This has not been true in drug development. Why? - the technical risks, regulatory overhear, and other parts. Can software enable monopolies in drug development?
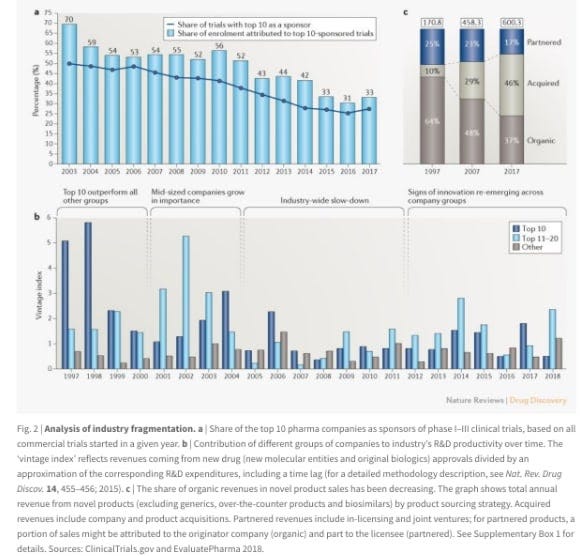
Source: Nature
How machine learning really impacts drug development
From a business perspective, understanding how machine learning creates a unique pipeline is going to be the difference between companies that bring medicines to patients over decades and the ones that burn endless amounts of capital.
Drug development involves a series of steps if executed properly results in a medicine that helps patients and most of the time billions of dollars. So very few industries have such market clarity. The hard part is that drug development is like running across a tightrope over a volcano with sharks with laser-beams on their head swimming around:
Select a disease ideally with high unmet need
If doing a mechanistic study, identify the mechanism-of-action driving the disease pathology and finding a suitable target to drug. If doing a phenotypic screen, go to step 3 and revisit step 2 later.
Screen for new molecular matter to find drug hits
Develop that hit into a drug-like compound by doing various preclinical studies - getting a sense of the drug’s efficacy and toxicity. Better and better models that map this preclinical data into human clinical data is a key driver for success.
Initiating an IND to enter the clinic, first showing safety then engaging in a pivotal trial to show efficacy
Each of these steps have a set of probabilities and costs associated with each - https://www.nature.com/articles/d41573-019-00074-z and https://www.bio.org/sites/default/files/legacy/bioorg/docs/Clinical%20Development%20Success%20Rates%202006-2015%20-%20BIO,%20Biomedtracker,%20Amplion%202016.pdf
Clinical exemptions like Orphan Drug Designations (accelerated approval, breakthrough designation, fast track, and priority review) can speed up the process and might boost the odds of success. Biomarkers are useful proxies but historically don’t produce clinically functional endpoints to drive approval but could help selecting hits and leads earlier in the process.
Machine learning can help for preclinical and clinical work:
Preclinical: work here roughly takes $30M-$60M and ~5 years (https://www.nature.com/articles/d41573-019-00074-z) and let’s see where machine learning can speed things up or lower costs
Traditional method
Target selection and hit generation : $2M-$3M
Hit-to-lead: $2M
Hit-to-lead time: 2
Lead optimization: $30M
Lead optimization time: 3
Machine learning driven method
Target selection and hit generation : $0.5M
Hit-to-lead: $1.5M
Hit-to-lead time: 1
Lead optimization: $20M
Lead optimization time: 2
These are just rough estimates, I would need to build out more formal models to validate the point, but simply machine learning can reduce preclinical development times by maybe around 40% and costs by ~44%. That’s interesting, but the next section will show that clinical costs get into the $100Ms - $20M here and there might not matter unless you can really increase the odds. This generation of machine learning drug companies will allow us to collect this data.
Clinical work here roughly takes ~$200M and 4-7 depending on the timing of the pivotal trial. There is usually phase I-III and submission work after a successful phase II/III. Machine learning could make an impact on improved patient recruitment and retention. Clinical design is likely to be the largest opportunity. Let’s say the impact of these new tools on clinical trials could be in line with the impact on preclinical work, ~40% of $200M is $80M - that’s the type of savings that can boost the total value of a given drug.
Food waste
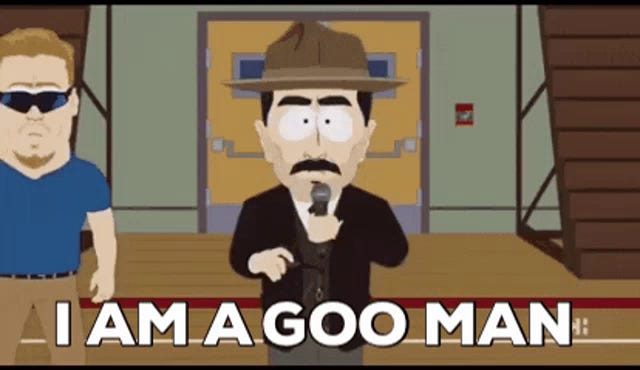
What’s the best way to solve food waste? Somewhere around 1.3B metric tons, that’s over 2800 pounds) of food is not consumed each year globally; that number probably is a lot higher - http://www.fao.org/food-loss-and-food-waste/en/ Like all forms of recycling the onus is put on the consumer where the producers have the highest leverage to reduce waste. However, the latter are not incentivized to reduce production and distributed more efficiently because that is lost sales.
In the US, at least 40% of all fruits and vegetables are lost at the farm and distributor level, not the consumer - https://www.nrdc.org/sites/default/files/wasted-food-IP.pdf This is due to the inelasticity of most foods - their price, with a diversity depending on the location the food will end up, often goes below the marginal cost of production. Looking at the cost curves of growers and consumers - food prices go up but growers are getting the same prices and probably less. Growers’ margins have been getting squeezed for decades. When a company like WalMart buys up over ¼ of all bananas, strawberries, and other foods, distributors regain the pricing power.
Food waste is a market incentives problem - the incentives of growers makes it attractive to overproduce and distributors have the margin to waste food.